LCM-Lookahead for Encoder-based Text-to-Image Personalization
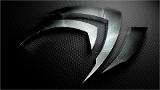
Recent advancements in diffusion models have introduced fast sampling methods that can effectively produce high-quality images in just one or a few denoising steps. Interestingly, when these are distilled from existing diffusion models, they often maintain alignment with the original model, retaining similar outputs for similar prompts and seeds. These properties present opportunities to leverage fast sampling methods as a shortcut-mechanism, using them to create a preview of denoised outputs through which we can backpropagate image-space losses. In this work, we explore the potential of using such shortcut-mechanisms to guide the personalization of text-to-image models to specific facial identities. We focus on encoder-based personalization approaches, and demonstrate that by augmenting their training with a lookahead identity loss, we can achieve higher identity fidelity, without sacrificing layout diversity or prompt alignment.